The Oxford dictionary defines fine-tuning in a way that sums up the idea perfectly: “making small adjustments to something to achieve better or desired performance”.
Today, fine-tuning in Generative AI becomes a key phase to obtain the maximum level of customization, and therefore, of value from this technology.
Basically, this is a technique in which foundational models, which have already been pre-trained with large volumes of “public” data, are customized with particular data that were not part of the training. This means tailoring them to more specific topics or narrowing their scope to a more focused target. To do this, you can take advantage of some solutions on the market, such as Amazon Bedrock.
LLMs (large language models) are trained with a vast amount of content. Fine-tuning in Generative AI allows them to be adjusted with data from the organization to produce results aligned with the needs of the business.
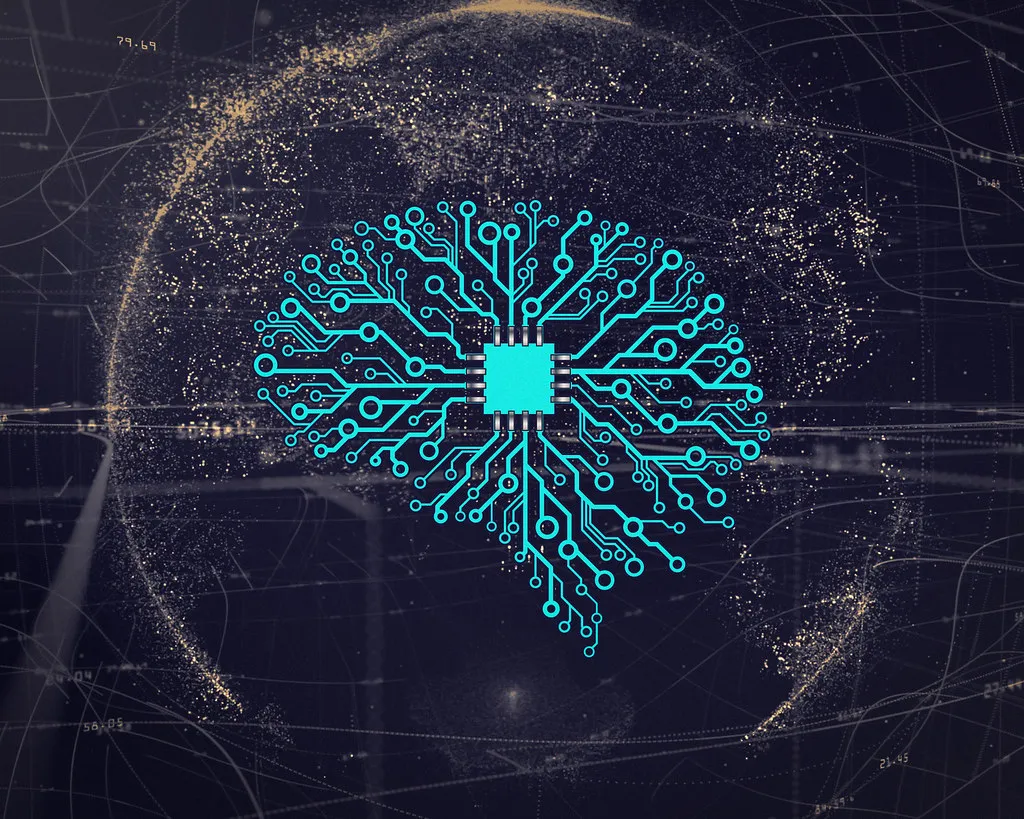
When fine-tuning in Generative AI is needed
To identify when fine-tuning is needed we must first understand what RAG (Retrieval Augmented Generation) is. It is the mechanism by which we can use an LLM with particular company data. Unlike fine-tuning, it is not through specific training, but through methods of searching and retrieving information from a corpus of data.
Although both methods serve to use a foundational model with the organization’s particular data, they are different mechanisms, with different limitations and requirements.
When do we really need fine-tuning? The answer is not easy, but there is a simple rule of thumb that can help in the decision making process. We will use it when we need to use an LLM with particular data and the results obtained via RAG are insufficient.
Other considerations to take into account are latency, scale and performance.
Fine-tuning, by its nature of having the model trained for a specific task, in general has lower latency than RAG.
In terms of scalability, both are limited by the size of the context that the foundational model supports. But by fine-tuning the context needed to solve a task is smaller than in RAG.
Finally, if the training has been done correctly, a better performance (accuracy-style metrics, F1 score, ROC AUC) of the model with fine-tuning than with RAG should be observed.
Benefits of fine-tuning pre-trained models
Fine-tuning in Generative AI offers numerous benefits:
Improves task performance:
Since specific training is performed to a pre-trained model, the model is aligned with domain-specific data, generating specialized knowledge. Thus, if the training was performed correctly, we can expect to have better performance metrics. In a way, it becomes adept at delivering accurate and contextually relevant results. This is fundamental for an organization, as it ensures consistency between the model and the business need.
It adapts better to the use case:
Each industry has its particularities, its characteristics and its specific data needs. The needs of a bank are not the same as those of a retail, telco or healthcare company. With fine-tuning in Generative AI, solutions are trained in a specific way and achieve more timely and effective results.
Reduces risks:
Helps avoid model hallucinations, as well as the delivery of incorrect, inconsistent or inappropriate information. Generative AI applications are mostly on the frontend (customer service, employee experience). This is therefore essential to avoid reputational or legal problems. With fine-tuning in Generative AI, responses are tailored to the needs of the organization.
How to perform Fine-Tuning in an LLM
As in any model training process, the first step in moving forward on fine-tuning in an LLM is to prepare the training and evaluation data set. In this respect, companies with a data culture will be one step ahead.
This is because it must be high quality data and specific to the task on which the solution will operate. It is important that it be a diverse and representative set, so that the model can learn the many nuances of the target domain.
How do you establish the size of that data set? Fine-tuning does not require a huge amount of data since it starts from a pre-trained model. However, good practice advises starting with a small subset for the first experiments. As results are obtained or as it becomes necessary, it is gradually increased.
The training itself consists of adjusting the model for the chosen domain from this data set.
The third stage is that of continuous improvement. With the fine-tuning process completed, the model is evaluated on the basis of a set of validations. This verifies whether it meets the expected performance criteria. If necessary, the process is iterated with the adjusted parameters in order to obtain even better results.
Regular evaluation of the adjusted model based on PKI (key performance indicators) helps to identify areas for improvement. These include accuracy, F1 score and ROC AUC.
Conclusions
Fine-tuning in Generative AI is a key process that allows us to customize pre-trained foundational models with our own data.
It enables better performance, higher levels of efficiency and better alignment with your specific business needs. However, it should be considered that this process involves training with trained data, with its respective software training costs.
In short: it is a very powerful mechanism that allows organizations to extract great value from their data powered by foundational models, through training to solve specific tasks with company-specific data.
Interested in maximizing your investments in generative AI? Our experts at Nubiral are waiting for your call. Schedule your meeting!
Te puede interesar:
Blog • Build and scale Generative AI applications with Amazon Bedrock
Blog • Amazon Bedrock: Innovation taken to the extreme
eBook • GenAI use cases with Amazon Bedrock